Intergeo 2017
Fraunhofer IPM presents the »Deep Learning Framework« for the automatic interpretation of 3D data
Using cameras or laser scanners to detect large structures generates vast amounts of data. For the first time, a newly developed deep learning framework is able to analyze this 3D and image data automatically. Fraunhofer IPM will be presenting the method at the INTERGEO 2017 trade fair from September 26–28 in Berlin.
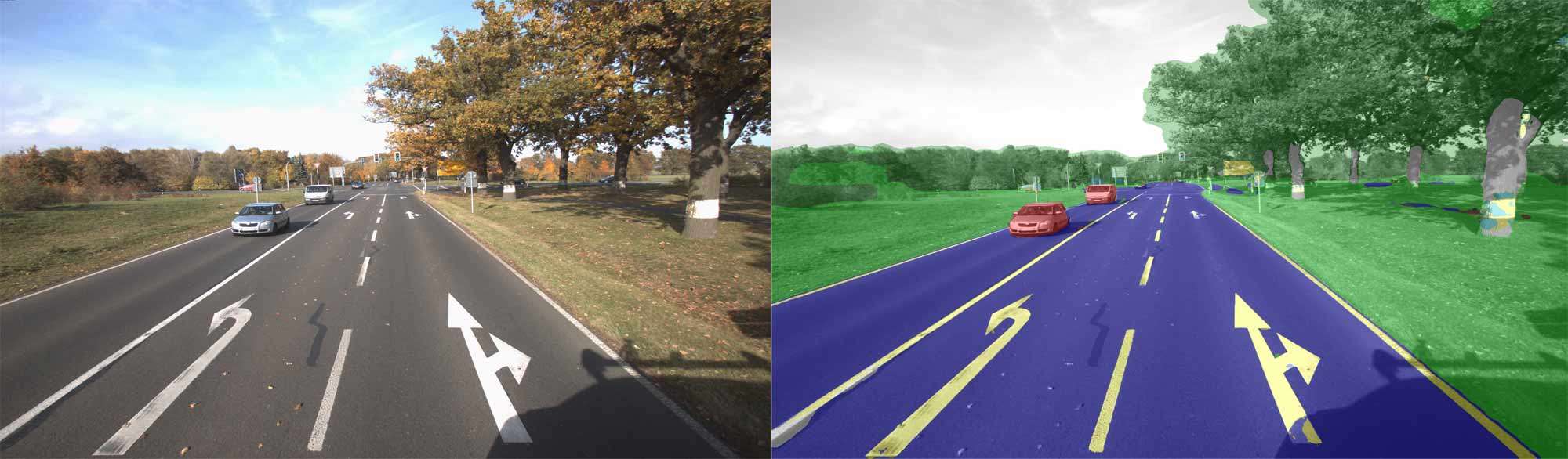
Monitoring the condition of large structures such as traffic routes, buildings or agricultural land creates enormous quantities of 3D and image data, which currently tends to be analyzed manually. This is time-consuming and costly. Using an approach which makes use of deep learning, Fraunhofer IPM has now succeeded in automating complex data analysis and interpretation. »Until now, data analysis has been a limiting factor when measuring large structures,« says Prof. Dr. Alexander Reiterer, Head of the Object and Shape Detection Business Unit at Fraunhofer IPM. »Rapid and cost-efficient data analysis, which is carried out automatically, will significantly expand the area of application of 3D data detection in the future.«
Real time evaluation thanks to artificial neural networks
As a method of machine learning, deep learning is a subfield of artificial intelligence and relies on smart algorithms. Training data sets are used to identify and classify objects, for example face recognition in a picture. In the deep learning framework developed by Fraunhofer IPM, learning algorithms are now able to evaluate 3D data automatically. The approach is based on artificial neural networks (ANN). In comparison to classical methods of object recognition, the deep learning framework has been shown to be more robust when confronted with the varying forms and obscured, damaged or faded objects that are typical of real-life scenes. Just a few years ago, it took weeks or even months to train such algorithms. Thanks to extensive parallelization, this process is now possible in just a few hours. New data sets can therefore even be evaluated in real time using a trained ANN. In ANNs, the information fed in passes through a multitude of interconnected artificial neurons, where it is processed and transmitted to other neurons. ANNs learn the output patterns which correspond to specific input patterns with the help of manually annotated training data. On the basis of this »experience«, new types of input data can then be analyzed in real time.
Data fusion: Merging scanner data and camera images
Camera or scanner data, as well as fused data, can provide the data pool for the automated object recognition. In the framework developed by Fraunhofer IPM, the georeferenced scanner data points are initially transferred to a grid format containing depth information and are then linked with RGB camera data. If high quality scanner data is available, it can be directly integrated into the framework. Ultimately, the type of data available and the purpose will always determine which approach is most suitable.
Fraunhofer IPM at INTERGEO 2017
In addition to the deep learning framework, Fraunhofer IPM will also be presenting mobile laser scanning systems at the INTERGEO 2017 trade fair in Berlin (booth D4.041): the Clearance Profile Scanner (CPS) for detecting surroundings from vehicles, and the Lightweight Airborne Profiler (LAP) which has been specifically developed for use on aerial vehicles.